There has been much written on the topic of ethics in artificial intelligence and innovation recently.
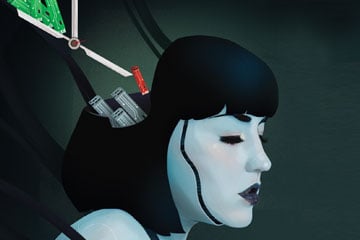
There has been much written on the topic of ethics in artificial intelligence and innovation recently. It seems we’re moving in the right direction in having an open discussion on the role of ethics and privacy in the algorithms and systems we rely on and use.
From what I’ve read so far: (1) Today’s AI and innovation development efforts are still in their teenage years, and (2) Trying to simplify the more complex human interactions and behaviours down into a bunch of mathematical equations with this early-generation technology is going to be messy and flawed.
According to one revered AI computer scientist: “We have more to fear from dumb systems that people think are smart than from intelligent systems that know their limits.” And as Meredith Broussard’s book Artificial Unintelligence: How Computers Misunderstand the World has it: “Just because you can imagine something doesn’t mean that it’s true, and just because you can imagine a future doesn’t mean that it will come to be.” (Does that mean no jet-packs then?) Her book deep-dives into several real-world examples to show the risks of too much techno-chauvinism — the belief that technology is always the solution.
The stories of people apparently blindly following Google maps into lakes and down one-way streets have been replaced with stories of professionals taking the outputs of sentencing and parole algorithms as fact. But there’s no magic in those algorithms; there’s no ghost-in-the-system coming up with the perfect answer free from human bias. Human bias is built into the very foundation of these systems. The data needed to train the algorithms is all socially constructed and is, therefore, flawed because of it. It is this bias in the data that points to a gap in how we teach the robots about the complexity of our world.
In the 2018 book The Book of Why: The New Science of Cause & Effect by Judea Pearl and Dana Mackenzie, there is a fascinating discussion about the predictive abilities of current AI and the modelling in high-frequency and habitual circumstances of Big Data. But the limits of this narrow AI mean it cannot yet handle places where learning systems are “governed by rich webs of causal forces.” Predicting how a judge might rule based on prior common fact scenarios is a great example of this narrow AI, particularly if it’s based on the clear “human-in-the-loop” algorithm training that companies such as Blue J Legal adopt. But predicting the recidivism risk rates for convicted criminals is different and needs a way to program in the complexities of our world. There isn’t yet an established way to remove the existing inequalities that get amplified using our current biased data sets. Data-driven decisions will never be 100-per-cent correct because they can’t yet take account of the randomness and nuance of real life. So, before we get too carried away with building algorithms that make decisions for us, we should be giving voice to the concerns of machine bias and the lack of an ethics framework or consideration of the socio-economic factors involved.
The classic ethical dilemma of the “trolley problem” and how it applies to the engineers designing and building self-driving cars is a case in point. How should cars choose between swerving to hit one person and instead hitting five or, indeed, killing its driver and passengers? Broader society needs to take a more active part in the design and build of new technology that will forever change our lives. Dr. Ann Cavoukian’s “Privacy by Design” framework, which builds privacy assurance by becoming “an organization’s default mode of operation,” may be a useful guide. It would embed references to broader social concerns at the very point of programming. In addition, ethics modules are now being built into computer science courses at universities. Deeper dialogue about the social impact of AI and its possible unintended consequences are beginning to take shape. And lawyers, philosophers, data scientists and others should all have a voice in designing our digital futures.
The role of lawyers?
Most of the legal tech AI on offer specifically denies that the algorithm is giving legal advice. The disclaimers help vendors avoid claims of unauthorized practice; lawyers are still “in the loop” in interpreting the outputs provided by the algorithms as part of their broader advice. If lawyers are to use AI to crunch through past decisions to help calculate risk or options for their client, they need to understand the reasoning that is going on in that black box. A more thorough understanding of the algorithm’s continuing education is also key. How is the algorithm learning and improving? What is the quality of the feedback and correction it is given and by whom?
Perhaps there is a further role for lawyers, too, as discussed at the Legal Innovation Conference at the University of Alberta’s Faculty of Law (hosted by dean Paul Paton) in January. In-house lawyers, compliance officers and their external counsel should also act as the conscience of their companies/clients and advise on the ethical as well as legal implications of the goods or services produced. I’m not sure where I sit on that, but, like the lawyers using this new technology on behalf of their clients, I think there is a responsibility to ensure AI-generated results are appropriate. This will involve digging into the data collected, the quality of that data and the flaws that a statistical model will have in its decision if seeking to solve genuine human or social problems.
A computer won’t always get things right; good old-fashioned paper-and-pen solutions with human oversight may be just perfect. And just because we can doesn’t always mean we should.
Kate Simpson is national director of knowledge management at Bennett Jones LLP. Opinions expressed are her own.